Centaur Stage is a weekly video series where we explore bold ideas about the future of work and learning. Each Thursday afternoon, on LinkedIn Live, at 2:30 PM UAE-time, Marilyn Zakhour, CEO & Founder of Cosmic Centaurs is joined by some incredible guests to share insights, opinions, and perspectives about the future of how we work and learn.
In this episode, the discussion between Marilyn and Phanish Puranam is centered around data and specifically organizational data, how it impacts the structure and design of companies, and the decisions that we make around them. Watch the full episode here.
About Phanish Puranam
Phanish Purnam a Professor of Strategy, and the Roland Berger Chaired Professor of Strategy and Organisation Design at INSEAD.
Phanish’s research in organizational science focuses on how organizations work, and how we can make them work better. His current interests include non-hierarchical organizations, the design of informal organizations, and organizational architectures that support self-assembling teams. Besides publishing his research extensively in peer-reviewed journals, Phanish has also written several books including:
“The Microstructure of Organizations” (Oxford University Press, 2018) offers researchers a new perspective on organization design.
"Corporate Strategy: Tools for analysis and decisions" (co-authored with Bart Vanneste, Cambridge University Press, 2016) which is used as a reference in MBA programs around the world.
“India Inside” (co-authored with Nirmalya Kumar, Harvard Business Review Press, 2012) won critical acclaim for its balanced look at the prospect of India emerging as a global hub for innovation.
Phanish obtained his Ph.D. at the Wharton School of the University of Pennsylvania in 2001 and was on the faculty of London Business School until 2012. Reflecting his commitment to doctoral training, he has served as the Academic Director for the Ph.D. Program at both London Business School and INSEAD.
About the topic
The global pandemic and the ‘great remote experiment’ have left many executives and teams reflecting on their work models, their structure, and their culture. As distributed and flexible work becomes more prevalent among organizations, we also have a unique opportunity: we have and can collect more data than ever before about teams and companies.
All this new data and analytics can help us at 3 levels of sophistication. These are what Phanish refers to as the 3Ps in his ebook. We can now Perceive or understand the current behaviors, Predict what they may look like and the third P, Prototype, or model and test how new decisions would impact organizational design.
To open the session, Marilyn shared a quote from the preface of Phanish’s recent ebook: The Organizational Analytics eBook: A Guide to Data-Driven Organization Design.
“Knowing how to organize may be humanity’s greatest and oldest accomplishment. But our lives today are also influenced by another more recent human achievement: algorithms.”
Before going deeper into the structural principles of organizational design and how new data can be used in an organization to do everything from allocating work, to integrating outputs and of course, making decisions about the future, Phanish takes us back to the fundamentals of what it means to design organizations.
“So, if I think about organizations, I'm just thinking about any group that has a goal. That could be a team of a few people, it could be a department, it could be a division or an entire company. I'm quite agnostic about thinking about all of those as organizations because regardless of the scale or what they do, all organizations have the same basic set of problems they have to solve. These basic problems revolve around division of labor. How do you convert the work into something that can be broken down into integration of effort? How do you put things back together again? That's so fundamental that it's going to be the case that you have to deal with this in all organizations across all sectors. So that's where we start.
I think where the data comes in is, rather than rely on intuition or copying best practice, we should try to actually find solutions to these fundamental problems that are context-specific and that work for your company or your organization. So that's really the way we want to bring these two ideas together, the problems on one side, and the solutions that have to be context-specific and we can use data and algorithms to find good options.”
Data and the pandemic effect
Marilyn starts by asking about the kind of data available. "Can you tell us about the new organizational data that is now available to us? This can be data that either has been made available because of the pandemic or data that has increased in accuracy that we can now focus on in order to make decisions."
According to Phanish, the data in people's space has always been lagging in terms of markets, customers, finance, accounting, or supply chain. He says, "the kind of data we had was personal records, how people progress through the system over time, whether they meet their KPIs, whether they're getting promoted, or how much they are getting paid. We had some data on things like networks and some further interview-based or survey-based data on culture. Broadly speaking, these were our sources of data."
He continues, "now what has changed? One simple fact. The pandemic forced all of us to work remotely and working remotely means working digitally, which means you leave a digital trace of the same activities that you were doing earlier with no trace. Now, because most of our interactions are happening on platforms like Zoom, Slack, or Microsoft Teams or things like that, effectively we have an electronic record of the way the organization actually works. That's been like a complete firehose of data, which we have never had in this field. Just in the last year, organizations have gathered more data about how they actually work than they probably ever had in their history. This is the golden opportunity that we were all waiting for because we had the ideas, we had the algorithms, we had the technical skills. We didn't have the data. Now we have the data. That's what makes it so special.
“Lots of stuff that people assume could happen in the corridor or in an informal conversation now has to be documented. While there are cons, one of the pros is that data quality has improved enormously compared to anything in the past.”
Perception, Prediction, Prototyping
Perception
Going back to Phanish’s ebook and his quote about how algorithms can help us in perception, prediction, and prototyping, he takes the example of HR issues inside an organization.“Let's take the issue of diversity and inclusion. Does our company have a D&I problem? Now, this is a question that can be very controversial. You can get very politicized around it. You can get very hot and angry about it, but there's nothing like some data actually showing you what's going on. One of my former students from our MBA program has been running this program at Grab where they went through this exercise of actually looking at the data and trying to understand where the imbalance is. At what levels in the hierarchy do you start seeing an imbalance between men and women and why? There's nothing like a ton of data to take all the passion out of the discussion and make it a rational, scientific decision."
Prediction
"Because for the most part, most people want to improve, it's just that without good data, you can't have that conversation. This is what I call perception. To be honest, you don't need more algorithms than what you see embedded in Excel. So this is table stakes. This is big data. What's new now is using big data to go beyond perceiving what's happening to being able to make predictions. That's where machine learning comes in. Machine learning is a prediction technology and using this data, we can make a prediction like who's likely to quit the company in the next 12 months? Who's at risk of attrition? Who's likely to do better if placed in a particular role? Which teams are likely to function better based on how we compose them? These are forecasts in some sense, and like all forecasts, we make them based on the theory that the past is sufficiently like the future to be able to make this leap. That's not always a good assumption, but often it's the best we have."
Prototyping
He continues, "Finally, the cutting-edge here is not really machine learning. The cutting edge is prototyping. The idea here that we can do A/B tests. Just like in medical trials, through randomization, we can have treatment and control groups. We can try it in one group and leave the other group untreated, look at the difference. Then we know for sure that if we see a difference, it must have been because of the intervention, right? To design these kinds of studies, the traditional way to do it is to do it at a big scale and use a lot of randomization and that creates its own political challenges.
Machine learning can actually help us to create what I call almost experiments. This is not really an experiment in the sense that you don't truly randomize. What you do is you find people who are statistical twins of each other. So let me give you a concrete example. I want to figure out, is there value in sending somebody to INSEAD for an Executive MBA. Let's assume that a client company is making this call, and is paying for this. How do they know? In large organizations, one way you might do this is to run a randomized control trial. You take 100 managers who are eligible to go, send 50 of them at random and compare the career progression of these 50 with the 50 that didn’t go. Now you can see immediately how controversial this is going to be, right? This is a non-starter for most companies. What we can do is we can send whoever wants to go and is available to go and so on, and then use the models that we have to find their statistical twins in the company who didn't go. These are people who on average are the same gender, the same age, the same career trajectory. So that becomes a control group. What the algorithms are doing is finding matches between the two. I think each of these examples shows you what one can do with data which you couldn't have done in the past.”
Designing experiments for organizations
Following this explanation by Phanish, Marilyn asked about the process of designing experiments for organizations and what the best approach is to negotiate the politics around running these experiments, and how these organizations communicate about them.
Phanish responded by saying that one way is to actually run a proper experiment and get approval from people at the top to do it and it is a very complicated process of getting approvals and sign-offs and so on. Another way is combining gamification with experiments.
“Suppose we have to decide between teams which are functional experts or teams which are composed of cross-functional experts. Which would be better in our context? Now I can show you a hundred academic articles arguing the first is good and I can show you another hundred arguing that the second is also good. And they're all right. Just right about different contexts, right? In your context, which is the right way? We'll never know until we run the test. How do we do it? We could run a full-fledged experiment but that would be costly. The alternative is to find a task that these teams do, which resembles what they're doing in real life, but in fact, can be done in a couple of hours. That's the gamification part. So suppose these teams are doing claims processing, for example. We should be able to find a mini case, which involves making a claim processing decision, which you can do in two hours. Now in real life, you might spend a day on it, but now you can do it in two hours. Once we have this many tasks, we can actually create a gamified environment where we take 50 teams, put them in the functional structure, take 50 other teams, put them into the divisional structure, run the same task, run it in the morning before lunch, and by evening we have the results. So this is the idea of thinking of organization design using a wind tunnel analogy.
After a discussion about training organizational design experts at the university, Marilyn asked Phanish about three tips he would give to data scientists or people looking at such data.
“One is about perception, you need to ask yourself what data am I not seeing? The problem is the things that get measured get focused on, but it could be that there are important issues that are not in the data because the data wasn't collected that way. And you'll never come to know. So what are the dark spots in the data?
Concerning prediction, this is where the risk of being late is particularly high right now because the technologies have moved very fast and most people don't understand them. People can come and tell you, we have a 92% accuracy rate in our model that makes predictions on who's going to exit. That sounds impressive until you realize that the turnover rate in the company is actually 8%, which means that if you just predicted nobody quits, you're only going to be wrong 8% of the time. That means you will have a 92% accuracy rate. You don't need a model for that. Knowing what it is that the model is adding beyond what we already knew how to do. That's the crucial question. You've got to set the horse race between what we'll be doing with human judgment versus what they are good at doing. Unless you run the horses, you can't really tell if you're winning.
Similarly, with prototyping, a lot of the experimental trials in the corporate world use the term experiment, but they don't have randomization. If you don't have randomization, I'm sorry, it's not an experiment. One of the worst ways you can run an experiment is the following. Let's say you have a new initiative and you want to pilot if it's going to work. The people who get to pilot it are the people who are the most enthusiastic about it. They sign up and you say, okay, go ahead and pilot it. This is the absolute worst thing you can do. The reason is these guys will do well, but they're not representative of what's going to happen when you run it out. So it has to be randomized. I think each of these are fairly common pitfalls.”
Time & Measurement
Marilyn then talked about a four-day workweek experiment which lots of people were excited about at the beginning but after 6 or 12 months of testing, the enthusiasm had toned-down, and a lot of the indicators that had been rated very positively, suddenly stopped being such a big differentiator. Marilyn asks, "how many years of data should an organization have or how long is considered enough in human behavior?
To answer this question, Phanish gave two examples of projects at Google. One is called “Project Oxygen”, which is designed to measure the impact of good managers and help the company train more of them. They first defined eight qualities of a good manager, which have become 10 now, and they ended up updating that every four or five years and they use this now across the entire company, all around the world to evaluate every manager and it affects their pay. So when you start consequential and it starts global, it's got to be up-to-date."
Google also runs A/B tests on deciding what food to put in which part of the kitchen. Phanish says, "They have all these work kitchens and they actually ran A/B tests to figure out how they can put the high-calorie stuff at the bottom so that it discourages people from snacking on unhealthy foods. That's obviously going to change if you have a bunch of people in their twenties and people who are in their forties or fifties. For me, if something was on the lowest shelf, I'm not going to touch it. But somebody much younger might not be deterred. That has to be something that you tune at a much faster frequency. It's really going to vary question by question. There isn't one generic concept.”
Organizational redesign following the pandemic
Marilyn asked if Phanish has worked with organizations that are using data and algorithms for an organizational redesign following the pandemic. Phanish said that he worked on two projects that are confidential, one is in the education field and another one in life sciences. "They gave the team access to entire servers of data on Microsoft teams meetings, Zoom meetings, and email in a very rigorous process of data protection and confidentiality. The point of this is to use the data to figure out how the organization adapted to the need to work remotely, who are the central actors who kind of integrate different people together across geographies, who are the people who need to be co-located when you can go back to work and who are the ones who can actually continue to work in a distributed way."
“This is going to be extremely important going forward because I don't think any company wants to consider the default option anymore where all their employees show up all the time. That's just not on the table. The question is who should come in and rather than just make it based on alphabetical order or employee number or seniority or something you might want to say, which groups of people are coherent, who worked together and you can see that in the data. That's really the direction we want to work towards.”
One last question
As with all guests, Marilyn asked Phanish to complete the sentence: The future of work is….
“The future of work is interesting," said Phanish. "I think we are standing at the cusp of some phenomenally interesting opportunities that can lead to some amazing outcomes. There are also some real risks that can lead to some really dystopian outcomes, right? Maybe I'll quickly highlight a couple. I think one can make a case that now that we've learned to work distributed, remote working should be the eco-friendly default option. That's the positive, the negative is that we might end up with organizations where people don't interact anymore face to face. The concept of an organizational culture then sounds archaic, right?
Having worked remotely means we work digitally. Now there are mountains of data that we've been talking about. We can use that to improve the way we work, or we can turn every company into a panopticon, where everybody's being monitored and surveyed to death. Again, I don't know which path we'll take. That's the reason I use the word interesting. There are two outcomes and in these situations and which one we adopt will really come down to leaders and their vision.“
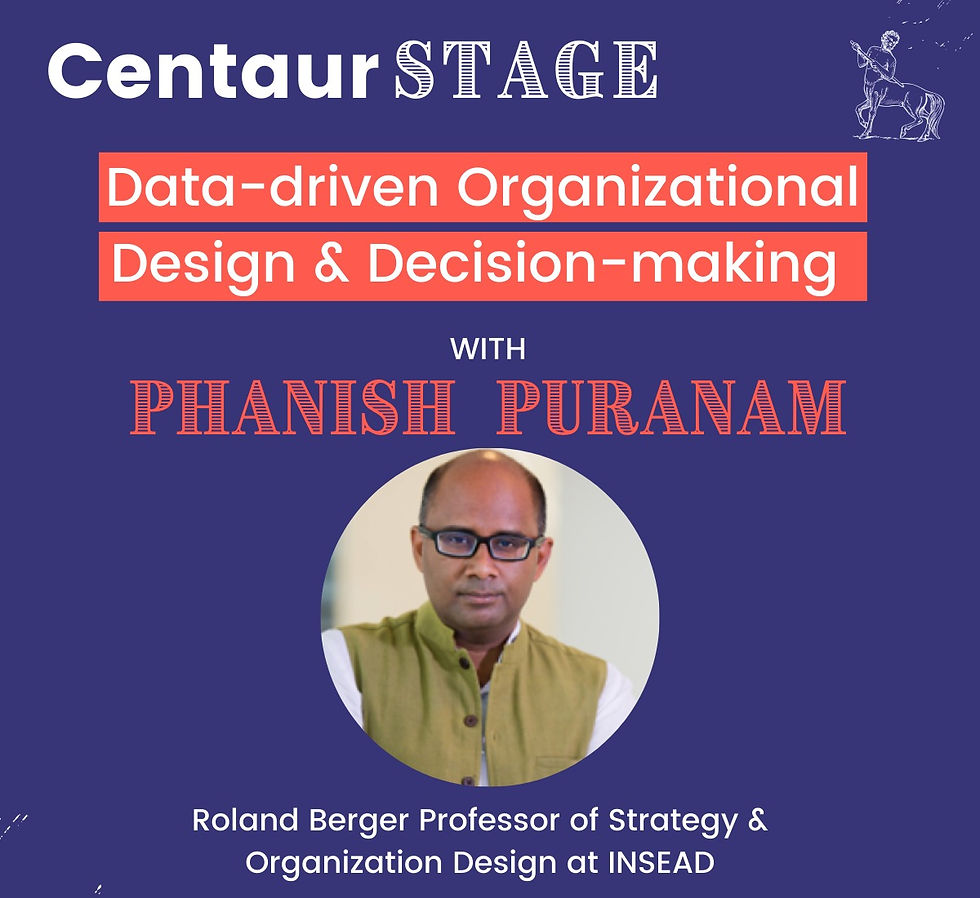
Other episodes of Centaur Stage:
Episode 6: WatchMarilyn's discussion with Nitesh Batra on mindful leadership or read the key insights here.
Episode 7: Watch or read the key insights from Marilyn's discussion with James Piecowye about the value of having range in your knowledge and skills, and how generalists will shape the future of work. James also talks about the interconnection of learning, and how this emerges in his teaching style.
Comentários